Manufacturing is being challenged at its core by multiple crises, but new technologies can speed its recovery — and increase resilience and flexibility — starting with quality management.
The COVID-19 pandemic has disrupted manufacturing and the supply chain deeply, impacting sourcing, logistics, production, and quality along the entire chain. The crisis has created new quality challenges, revealing weaknesses in existing quality-management (QM) approaches and bringing manufacturing resilience into sharp focus. In this context, digital approaches to QM are becoming even more relevant: By leveraging advanced analytics and automation technology, digitization can achieve greater efficiency, resilience and will provide a fast and lasting impact.
Three digital dimensions of quality management
Most companies have yet to achieve full digital transformation, but with challenges for manufacturers and the need for resilience on the rise, QM can be a successful driver on the road toward technological evolution. Industry 4.0 comprises various digital technologies — from connectivity, to advanced analytics, robotics, and automation — all of which are on the way to revolutionizing QM in the next five to 10 years. Some of these digital solutions are already being successfully deployed and have been shown individually to lower the cost of non-quality from 10 to 20 percent. And a specific menu of digital quality management solutions can lead up to a 50 percent reduction, creating enormous opportunities for change. To become more digitized, automated, and distributed, manufacturing firms must incorporate and leverage three dimensions in their operations: digitally enabled production and quality feedback loop; AI-based quality testing and vision control; and automated, real-time production and quality control tower.
Digitally enabled production-and-quality feedback loop
Production is often disrupted in labor-intensive processes and related manufacturing industries, affecting overall performance. Fluctuations may be driven by labor shortages, lack of training, increased rework rate, and scrap rate, all of which directly impact the overall throughput and the total cost of non-quality.
Traditionally, manufacturers have tried to reduce those impacts by establishing quality gates on key production steps — a reactive process that is time consuming, not fully reliable, and triggers problem solving only in severe cases. Their efforts have been challenged by the lack of a data infrastructure to provide necessary transparency on real-time performance, as well as a missing data integration, thus leading to limited views — and potentially a misunderstanding — of actual production performance.
Digitally enabled production-and-quality feedback loops use advanced analytics and process verification in real time to track trends, prevent deviations or out-of-specification production, optimize rework, and remove bottlenecks faster. Employing digital tools that are easy to use and understand by operators and supervisors, the feedback loop translates work procedures into visual guidance and quality checks. The feedback loop also predicts negative quality trends before they turn into increased rework and bottlenecks.
One global component supplier implemented a tablet-based quality feedback loop at its lead plant in the Americas. In less than six months, the rework rate had fallen from more than 25 percent to less than 10 percent, removing production bottlenecks and achieving $35 million annual savings.
This innovative solution does not require a holistic Industrial Internet of Things (IIoT) platform or large investments. Instead, it leverages existing operations’ data in two aspects: first, by complex analytics and AI based algorithms providing the essential information needed to apply just-in-time corrections sequentially so as not to disrupt production workflow; and second, aiming targeted information and operational advice to line workers in an easy, convenient format.
It is about not disrupting the optimized workflow of the workforce built up over many decades, and in providing real-time, contextual instructions to ensure that the operation meets specifications and targets.
AI-based quality testing and vision control
Another challenge to quality processes is manual inspections, which typically require a large number of inspectors and take time. While quality checks are critical for ensuring the excellence of the product, they can give a false sense of security due to inspector variation, unknown defects, and human error.
Implementing a testing-and-vision control system along with a machine learning (ML)-based process and quality control on the production line can identify operator errors, quality deviations, and process variations in real time. The system processes inputs via multiple cameras, applies deep learning models for detecting defects and anomalies, and acts as a digital assistant by alerting the operator to issues and providing instructions for fixing the problems. Additionally, the AI-based vision control system feeds into a digital twin, providing real-time insights to industrial engineering, production planning, and quality management.
Several assembly-based industries, with multiple manual operations and procedures, have employed in-station automated production and quality control systems that have had the impact of cutting rework rates dramatically by providing direct, real-time feedback to operators, preventing many problems downstream.
Other benefits include cutting cycle time due to automated defect detection (up to 20 percent defect detection rate improvement) and reducing reliance on downstream end-of-line manual inspection. These systems can also provide visibility into lean improvement measures and productivity improvements and the efficacy
of implemented corrective actions for quality issues.
Automated, real-time production and quality-control tower
Even production and quality leaders have only limited real-time data and transparency into operations and quality performance in a single plant or across multiple plants. The challenges of managing a production ramp-up while contending with quality issues after delays, increases systemic stress and jeopardizes achievement of throughput targets. And, if there is no sharing of data between functions or production stations, then you are limited to hot spot improvements rather than end-to-end optimization.
A production and quality-control tower can tackle those challenges and enable preventive quality assurance. (See Exhibit 1.) The interconnected quality-management system comprises three crucial elements:
- Real-time operations monitoring, from single production-station level to multi-plant levelto allow quick deviations identification, problem solving and decision-making
- Advanced analytics-based alerts, prediction of performance trends, and actionable improvement insights to prevent larger quality issues landing further upstream
- End-to-end process performance for quality management and dependent functions by use of centralized data and cross-functional KPIs to provide a consolidated view on operations performance
For example, a global leading component supplier implemented the production and quality-control tower to make product and process quality accessible from product development through to delivery of the finished product. The implementation brought about a 17 percent reduction in manufacturing rework and 15 percent reduction in non-quality cost, as well as indirect production improvementsof 8 percent in overall equipment efficiency (OEE).
Exhibit 1: Quality control tower application interfaces (browser-based)
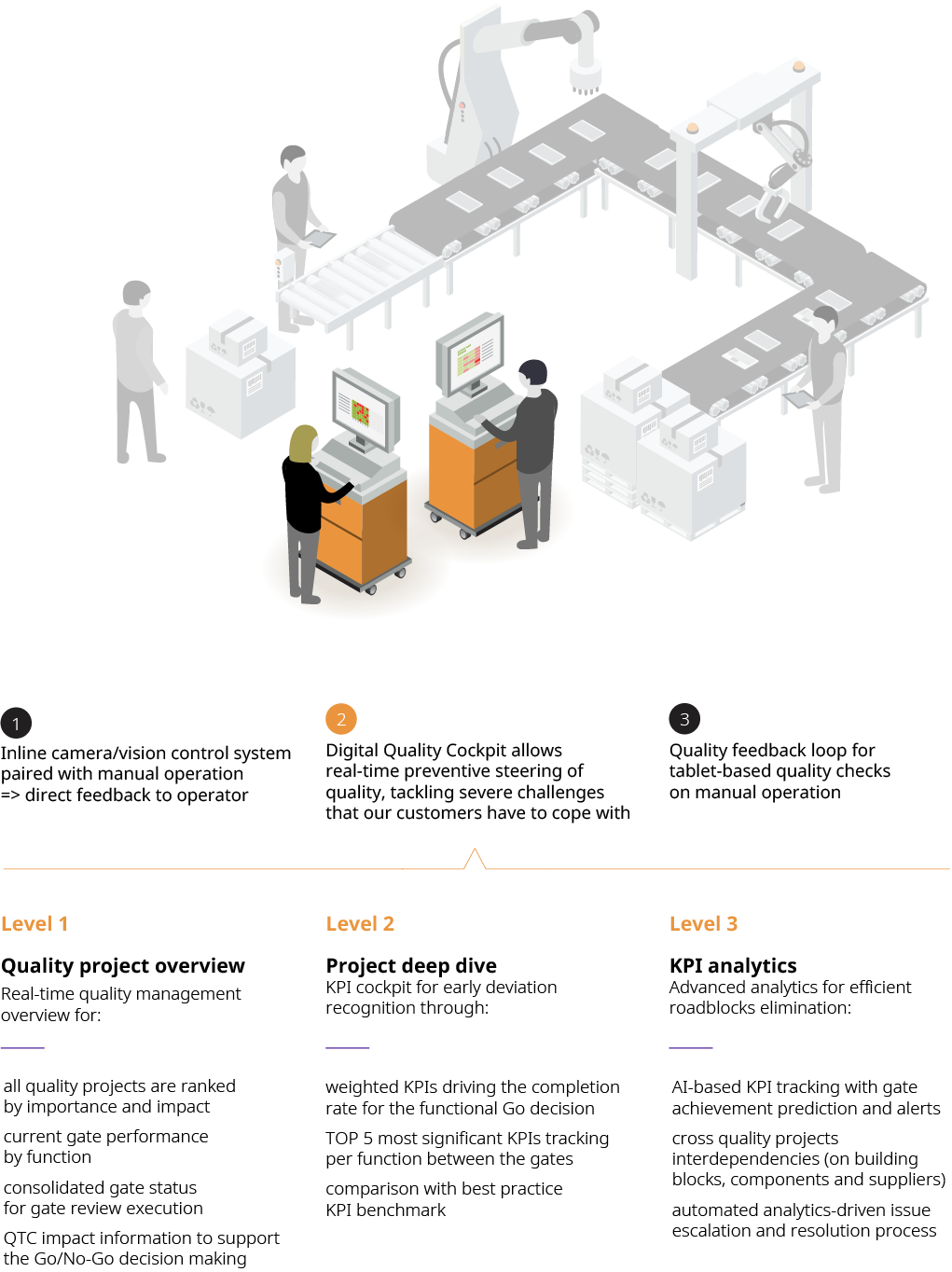
Source: Oliver Wyman analysis
Outlook
Manufacturing industries are likely to see continued uncertainty in demand as well as disruptions to the supply chain in the coming years. It is of the essence that manufacturers begin using digital solutions to build resilience. The technologies needed to attain the three digital dimensions of quality management already existing and are readily available. Implementing them will benefit manufacturing performance and improve quality in the near term. In the long term, it will result in greater operational resilience and fewer supplier-driven disruptions by preventing quality-related issues and reducing volatility when ramping up production.