Artificial intelligence (AI) and machine learning innovations are beginning to transform a broad array of business functions, including manufacturing processes, with promising use cases ranging from research and development to sales. However, to truly leverage the abundance of production data and make use of AI, manufacturers first must connect standalone AI use cases and create an integrated AI strategy, taking production through edge computing to the next level.
Artificial intelligence and machine learning are the buzzwords of the moment, promising to enhance business processes, quality, performance, and profitability. Indeed, AI-based technologies and applications are expected to conquer many functional areas within companies and industries in the years to come, manufacturing industries included. But the road to success for AI has yet to be paved. A question remains: How are the improvements promised by AI to be measured and quantified?
A HOLISTIC AI STRATEGY: INTERLINKING THE DATA
Since the root of most AI-applications lies in using and interlinking different data sources, manufacturing companies (with their abundance of different data pools, ranging from R&D to real-time production data to sales figures) hope to see significant improvement in performance by making use of artificial intelligence. AI is expected to impact the industry with a significant revenue uplift and a simultaneous productivity gain of more than 40 percent. Equipment effectiveness can be expected to reach levels close to 85 percent when being AI-enhanced. (See Exhibit 1.) But, in order to realize the full potential of AI applications, it is important to develop a holistic and integrated AI strategy. Without an overriding strategy, the AI use cases will become stand-alone solutions, yielding an inferior patchwork.
But if separate departments develop individual solutions, use detached data lakes, or employ their own legacy dataprocessing infrastructure, then AI’s full potential will never be realized. By developing an overarching approach to AI – an approach that recognizes that AI applications will sooner or later affect all areas of the value chain – and adapting a data-first state-of-mind, manufacturers can create a sustainable competitive advantage.
Exhibit 1: Overall equipment effectiveness of typical manufacturing companies
Source: Oliver Wyman analysis
EDGE COMPUTING: BRINGING AI TO THE DATA AND NOT VICE VERSA
The promise of AI, however, depends on more than just highly interconnected devices. Even if multiple data sources can be connected to generate meaningful insights, AI manufacturing applications require real-time data processing and the ability to act instantly on the insights generated. Thus, processing the enormous amount of data provided by an ever-increasing number of sensors in the production process requires edge computing, putting analytics right at the data source instead of in the cloud. Currently, most manufacturing firms resort to cloud computing or are still using their own local data processing capacities. But to create real value from the interconnecting hardware and production facilities calls for AI to be used where the data originates.
For instance, a production robot equipped with multiple data-generating sensors will be able to use machine learning models to predict a defect or malfunction in real time and be authorized to shut down autonomously to prevent damage. In the case of traditional data processing, the production data collected by the robot’s sensors would be sent to the cloud. The data analysis, as well as the order to shut down the robot, would have to be sent from the production facility to a server and back again. This would simply take too long, causing severe damage to the robot or the overall production process.
Thus, some AI use cases are only possible if deployed in real-time. Edge computing – computing that takes place directly at the edge of the data source, whether it be the robot or the production line – is a pivotal enabler for doing so.
THREE KEY APPLICATION AREAS FOR AI IN THE FACTORY
If the two principles of a data-first, holistic AI strategy and of bringing AI to the data source are taken to heart, then the following AI uses will allow manufacturing firms to get the most out of artificial intelligence and gain a competitive advantage. It is important to keep in mind that this is not a comprehensive list. Instead, the three uses are easy to implement and can serve as stepping-stones towards an AI-driven manufacturing firm.
First, machine-learning prediction models can improve planning and production scheduling significantly, moving from experience and gut feeling to fact-based dynamic planning. By connecting real-time production data with planning and sales information, an AI-system could learn from previous conflicts in schedules, launch ramp-ups in production, and independently adjust production towards quality capacity planning. Through the integration of unstructured data across the entire supply chain, machine-learning models could continuously adjust conflicting system parameters to optimize output/wear-and-tear ratios or provide real-time process solutions to control the end-to- end manufacturing process. By doing so, the planning and production could be lifted to a level of accuracy that is unattainable via traditional methods.
Second, leveraging stochastic methods, machine learning, and AI to predict key sources of defects and manufacturing problems will bring predictive maintenance and in-line quality management to its full potential. Through edge computing, Internet of Things (IoT) components in a production process will be able to detect defects and irregularities in real-time and adjust manufacturing steps accordingly. Enabled by machine-learning analytics, IoT components will be able to untangle complex, hard-to identify defects and quality issues and find sustainable solutions. Thus, manufacturers will be able to detect defects based on multidimensional attribute analysis and enable machines to not only detect and flag but to also solve the problem.
Third, an integrated AI strategy across the entire value chain will help manufacturing firms establish advanced supplier quality control and create an agile and adaptive supply chain. Being able to adjust supply not only allows manufacturers to adjust output to demand more easily, a modularized and IoT-network-based production process lets them satisfy the need for variety and customization. For instance, AI-steered production equipment allows producers to translate individual customer demand into 3D-printable output.
40% productivity gain through AI-use cases until 2025
THE AI-TRANSFORMATION PROCESS
To transition towards an AI-driven company and realize the full potential of advanced analytics, manufacturers must: 1) integrate different data sources; 2) gain speed through edge computing; and 3) avoid creating a patchwork of AI use cases and instead develop an overarching AI strategy for the entire value chain.
Despite the hype surrounding AI, it is important to start realistically, even when having a rather disruptive target picture. For instance, the creation of AI lighthouses can promote AI applications within the organization and help to gain experience and trust. This is particularly important when accounting for the fact that a lot of AI tools like convolutional neural networks or other deep AI techniques are black boxes when it comes to the underlying data processing. At the end, this is what makes true AI powerful: It surpasses human dataprocessing capacity.

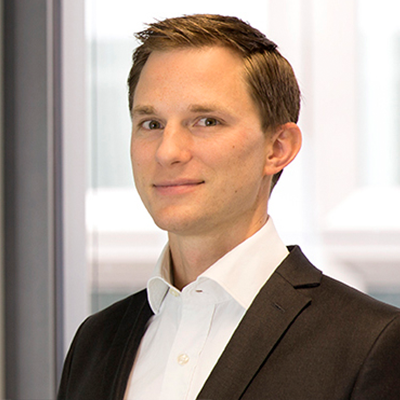