By: Michael Lierow, Cornelius Herzog, Stefan Blank, Eva Sprengnetter
This article was first published on May 8, 2020.
The COVID-19 pandemic has brought more than two-thirds of the world economy to a grinding halt. Many companies face a collapsing demand, resulting in severe cash-flow problems that ultimately might result in bankruptcy. In this situation, tight cash management has become the top priority for 2020 to ensure business continuity, especially as a lengthy recession will likely follow the immediate COVID-19 shock.
Companies need to immediately apply a set of actions to stop avoidable cash burn—such as reduced working hours for employees—but also think about more sustainable and long-term measures to free cash.
In many companies, a significant amount of cash is tied to inventory. Thus, an advanced forecasting and inventory-management approach is a key lever to free cash without sacrificing the service performance of the business.
Advanced forecasting and inventory management
To sustainably reduce inventory levels, businesses need to holistically optimize their supply chain— with better forecasting, updated inventory policies, and smarter procurement mechanisms. Based on our analysis, we have found several tactical measures that can reduce inventory by 15-50 percent, while also improving availability for their most critical parts.
Exhibit 1: Forecasting to cash approach

Machine learning (ML) based forecasting including overlays: Using an ML platform to predict future demand significantly improves forecasting accuracy compared to that of statistical-forecasting models. That’s because “hidden” patterns can be identified with large datasets. Moreover, based on new data feeds, ML algorithms will learn from shifting dynamics during the crisis and thus can better predict crisis-demand patterns than traditional models. During the initial learning period, manual overlays, such as human intervention, are needed to make sure the numerical results are making real-world sense (see Exhibit 2).
Exhibit 2: Superior accuracy of machine learning forecasting
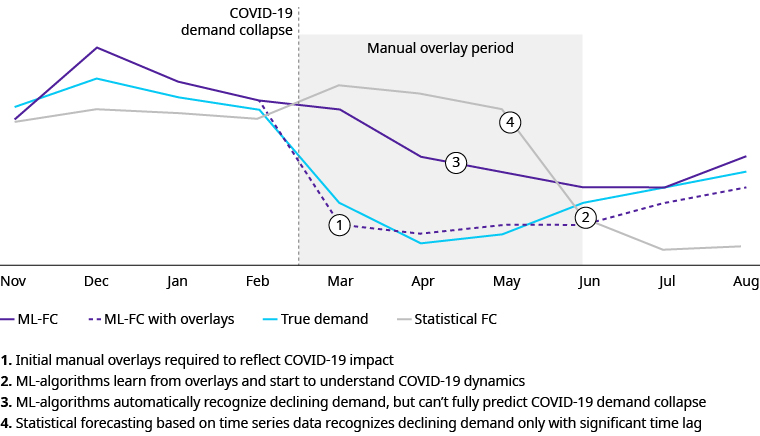
Dynamic inventory management: Replace simplified inventory-level calculations, such as minimum and maximum levels, with statistical safety stock using means and standard deviations to account for supply and demand uncertainty. This is a function of demand and supply variations, as well as targeted service levels. These can be differentiated by product clusters, customer groups, or crisis-related product requirements.
Order book re-alignment: Implement a procurement watchtower to review each purchase order and ensure a cost-optimal quantity is purchased. For example, it might be cost-effective to buy 1,000 parts at once, but negotiating with the supplier to deliver—and pay for—four batches of 250.
Track and trace: To ensure sustainable impact of the approach, it has to be continuously monitored using a comprehensive set of key performance indicators (KPIs), including forecast accuracy, number of canceled orders, inventory burn rate, and service level performance. In case of deviations, corrective actions can be implemented immediately.
If done right, the “Forecasting to Cash” approach offers significant potential for businesses across various industries. It allows to burn unnecessary inventory of low-risk parts to release cash and increases safety stock for high-risk parts to secure their availability (see Exhibit 3).
Exhibit 3: Impact of advanced inventory management
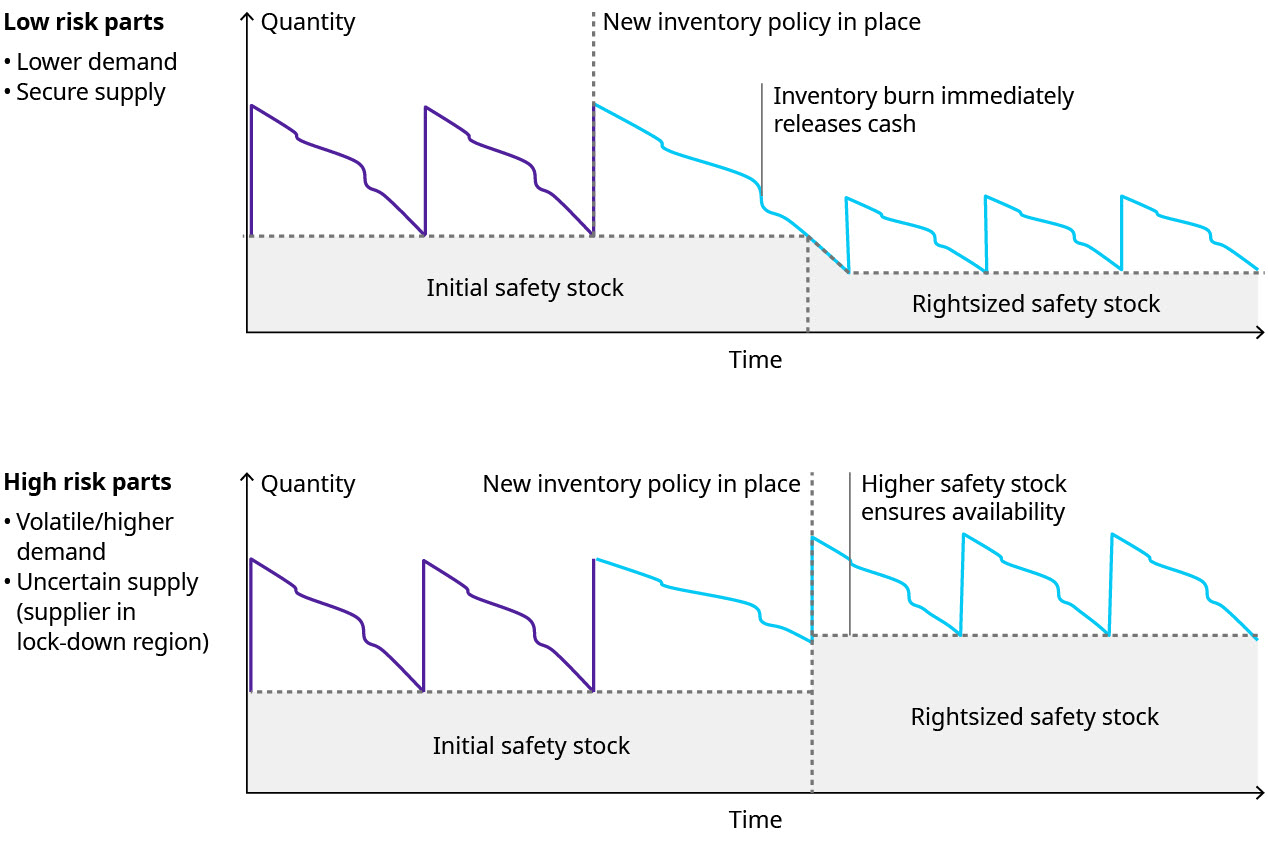
Depending on the maturity of the forecasting and inventory management in place, these measures allow to reduce inventory for low-risk parts in the range of 10-50 percent, resulting in lower working capital and inventory carrying cost. Simultaneously, the rightsized safety stock allows organizations to better fulfill the demand for high-risk parts resulting in revenue upside of 2-10 percent.
Given the potential on hand, businesses should react now and quickly tackle these hidden upsides. The key to sustainably realizing impact is to refine the entire “Forecast to Order” ecosystem. Besides implementing a new ML-forecasting algorithm, this includes the redesign of planning processes and policies, such as demand, supply, order policy, as well as the implementation of a tailored, KPI-based, monitoring system and corresponding governance.

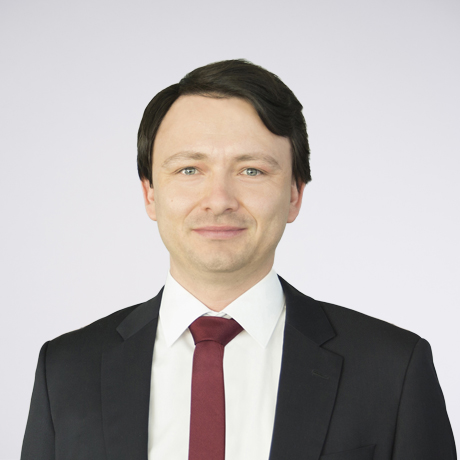
Cornelius is based in the Munich office.
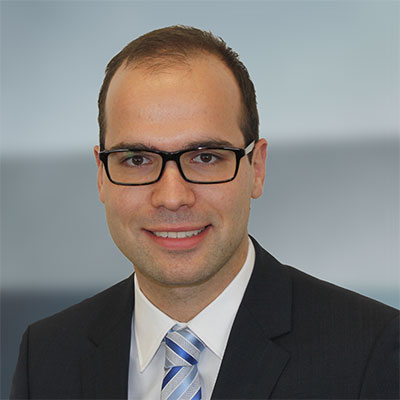
Stefan is based in the Munich office.
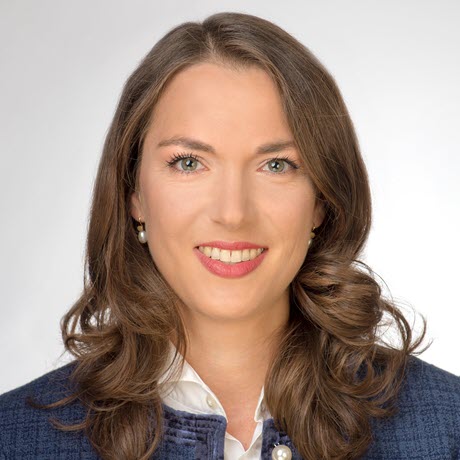
Eva is based in the Düsseldorf office.