The operators typically order in bulk from the manufacture—say, a couple of months’ supply at a time—so they can get a discount. However, it’s hard to predict how many they will need, and getting it wrong causes losses: Over-order, and the operator has to get rid of the excess at a reduced price; under-order and it misses out on sales, losing revenues and sending potential customers elsewhere.
Advanced forecasting techniques based on machine learning can significantly improve operators’ management of handsets, both overall and at a branch level. In a test run, a model developed by Oliver Wyman produced weekly forecasts that were between 80 and 85 percent accurate at the device level. This can help an operator boost device related revenue by up to 20 percent and reduce its working capital devoted to handsets by 50 percent.
These results are enabled by
- Selecting the right combination of variables into the model such as device metadata (e.g. release date, color, storage, sim type, screen size) and historical sales indicators (shifted sales, EMA, MACD, etc.)
- Deploying the right machine learning model that fits with the client context and data complexity—in a previous client situation, we tested several machine learning models and selected the XGBoost model (Random-Forest model with gradient boosting feature) due to ability to auto-improve gradually while building the trees one-at-a-time, which helps the algorithm to converge quickly towards the right forecast provided limited data noise (which can otherwise result in overfitting)
The forecasting methods are part of a wider adoption of analytics and big-data capabilities by telecom operators. So far, many use them to automate some network functions and personalize both customer experiences and advertising. For example, a customer’s past behaviour indicates the likelihood that they will leave for another provider and also provides clues as to what kind of incentive—a discount perhaps, or an allocation of free call minutes or data—will work best to retain them.
For handsets, operators have typically made forecasts manually, based on historical sales data in similar situations. They might prepare a weekly spreadsheet showing devices and sales over the previous week. These provide a reasonable estimate for sales in the following week, because the weekly variation is not usually that great. But such a method can only handle relatively simple data, so cannot take into account factors that might influence sales, such as versions in different designs, new device launches seasonal effects such as holidays, and the impact of promotions.
Exhibit 1: Sales in units for all SKUs
Week 1 2019
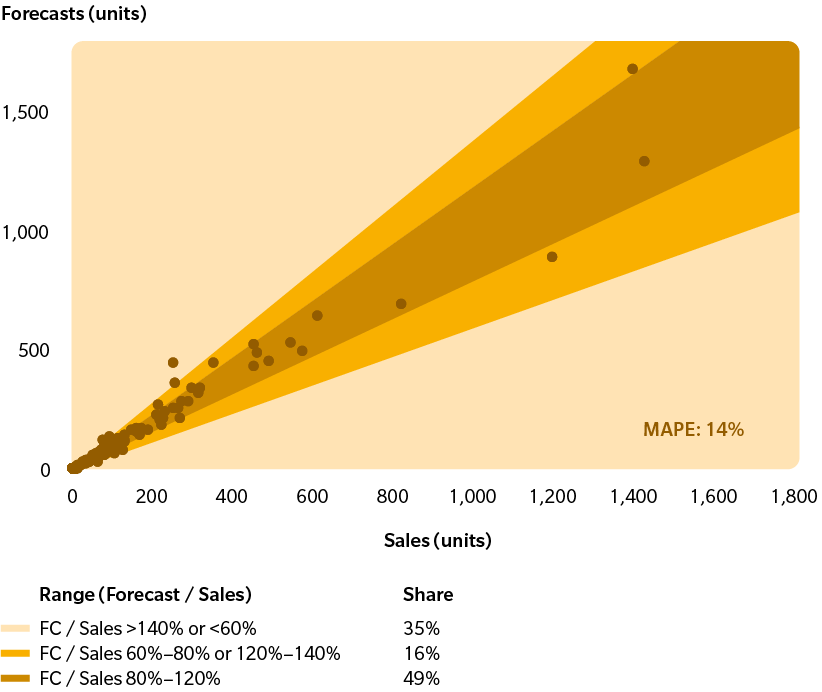
X Axis: Shows actual sales of week 1 in 2019 Y Axis: Shows what model predicted – dots represent different device types illustrated along the (X,Y) plot
With advanced forecasting techniques, an algorithm is trained on vast quantities of past sales and promotional data. It first identifies factors that might affect handset demand. Then the algorithm analyzes data on combinations of these factors—an expensive version in red, a cheaper version in green, or a silver version with extra storage capacity—to forecast future trends in detail. Model training takes just a few minutes and several forecasts can be conducted simultaneously. The process can be fully automated so that no manual work is involved. It is thus faster than traditional techniques, and its results are more accurate.
The objective with handsets is to forecast weekly sales so that the right number can be ordered from suppliers. For an order of a certain number of handsets, the model computes the potential financial loss from inventory excess if the order turns out to have been too large and they have to be sold off at a discount. It also calculates the downside that would result if the order turns out to have been too small. And the model works out the probabilities of various sales numbers. The operator can then choose the optimum order size to minimize potential losses.
However, while the operator might have ordered the right quantity on an aggregated level, demand fluctuates in its various stores, so some can end up with excess stock while others with shortages. The forecasting technique can help here too, calculating the right number of devices to distribute from the operator’s warehouse to its different branches and sales points.
Exhibit 2: Optimization of forecast quantities
In USD MM
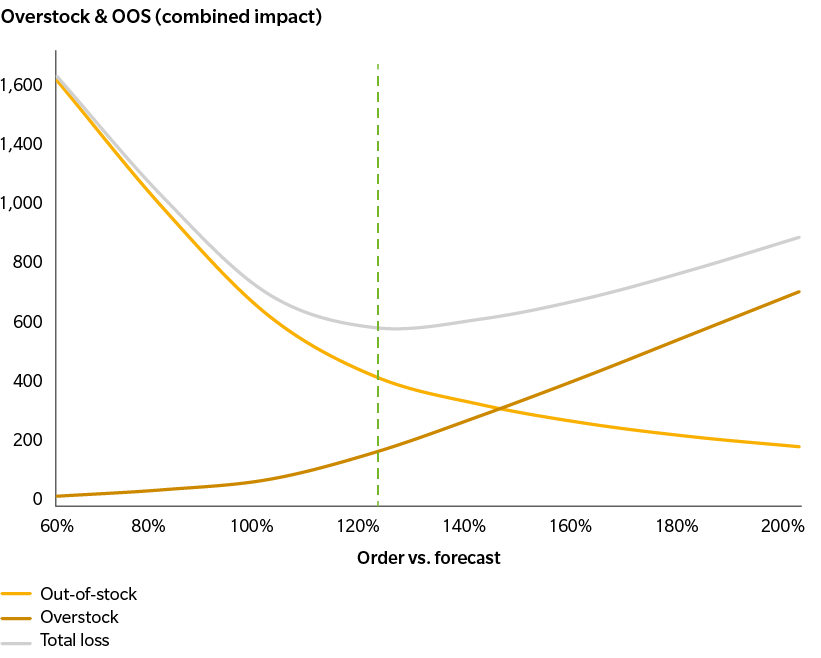
X Axis: shows the level of order we select vs. the forecast we obtain—e.g. 100% would mean that we order exactly our forecast
Y Axis: Shows based on the order magnitude vs. forecast, our Out-of-Stock loss (loss due to devices not available in the store), overstock loss (due to immobilized devices within the warehouse / stores) and combined effect (showing an inflection point when we order 120% of our forecast
Machine-learning techniques can help other important forecasts too. If operators have an idea in advance of the volume of data customers will use, they will find it easier to commit to buying wholesale volumes, which will help them negotiate better rates. Forecasts of demand for various spare parts over a certain period enable operators safely to reduce their inventories—as many parts will only work for a particular version of a smartphone, which will soon be out of production. Operators can also commit to buying a certain volume upfront, enabling them to negotiate a bulk discount.
Advanced forecasting requires buy-in from the senior management, not least because they will need to ensure the operator’s processes are redesigned to use it. Essential data requirements should be in place in advance—such as shipment information and stock levels, as well as historical sales and their relations to holidays, promotions, and other factors. Data skills are needed too, to manage potential changes to the model in the future. So telecom operators should start to get ready.