The increasing wealth of data available to the automotive industry has led to a large number of application fields for big data. Decision support based on data can be extremely powerful across the entire automotive value chain. Many critical decisions, however, are still being made without tapping the full potential of big data. For example, automakers on average directly spend more than 10 percent of the vehicle price on discounts (not including additional dealer discounts at the point of sale). Furthermore, the likelihood that a customer will purchase another vehicle from the same automaker is only about 50 percent across markets and brands. Both areas are of vital financial importance to the industry – but each is still only partially assisted by systematic data analysis.
There are a couple of reasons for this. First, only recently have automakers had access to comprehensive customer data from areas such as telematics, mobility services, improved customer relationship management (CRM), and stronger dealer integration. Second, there have been substantial implementation challenges. Systems nobody uses, data results that are not trusted, and reports that lack practical importance – the bottom line is that many big data initiatives fail due to misconceived implementation. Oliver Wyman has identified a number of factors that are key to increasing the chances that big data initiatives in the automotive sector will be successfully implemented.
Start With Decisions
In contrast to public opinion, big data analysis only gives answers to specific questions. The key is to start with specific decisions that need to be improved. Examples in automotive sales would be early contract termination, upselling of service products during the vehicle usage term, or profit optimized configuration of stock vehicles. It is crucial to understand which decisions drive value and then to determine the right analytics and intelligence to address these questions and improve results. Data provision and consolidation is a subsequent step.
For The User, By The User
It is often impossible to develop applications that truly enable better, faster, simpler decisions when applying traditional IT approaches such as waterfall processes and data warehouse programming. Why? Because tailoring fit-for-purpose decision support requires constant interaction with the final users. There must be a “for the users, by the users” mindset. That means prototypes that can be tested and criticized as well as agile approaches to programming that quickly turn the prototyping approach into improvements that excite. This typically requires an agile data layer – a so-called “sandbox environment”– at least for the development stage. Primary systems rarely need to be changed to accommodate a big data application because that would take too long. What’s required instead is an agile data mart that feeds off and integrates all possible data sources. Such an approach not only leads to better results but also to much shorter development times. Typically, the time-to-realization can be cut by a factor of three to six. That means that what in the past would have taken a year and would have included substantial usability risks can be done in two to four months, with better usability and relevance.
The problem is that many big data projects start out as large IT projects, based on hundreds of pages of theoretical specifications and programmed directly into the core data warehouse (which must support many core processes in parallel). Due to a lack of guidance from the actual decision-makers and because of superficially defined analytic outcomes, many projects often end up being over-engineered and fail to meet user needs. In the end, the applications will not be used and will be written off.
Big data analytics must be set up as learning systems. No single analytical project will have all the answers beforehand. What’s vital to achieving the desired results is testing on specific customer data sets, implementing actions, and reviewing them.
Design For The Decision-Makers
Big data applications are only effective if they are used by decision-makers for decision-making. The reality is that many decision-makers lack this access because it is often restricted to a few managers, due to perceived confidentiality issues or top management exclusivity. What is worse is when these milliondollar systems have just a single-digit number of users, because they are either little known or so difficult to operate. The effectiveness of widespread system access is evident. Decision-making in large corporations is – despite top management perception – a decentralized process. Many key decisions (such as discounting, ordering, engineering specifications) are made by a multitude of decision-makers spread throughout the organization. If those people are provided with the right analytics, decision-making quality will sustainably improve. In addition, widespread access will enhance a common understanding based on the same data. It is also crucial that applications are part of organizational processes. If, for example, a sales steering tool is developed, it needs to be defined as a standard element of the sales planning process.
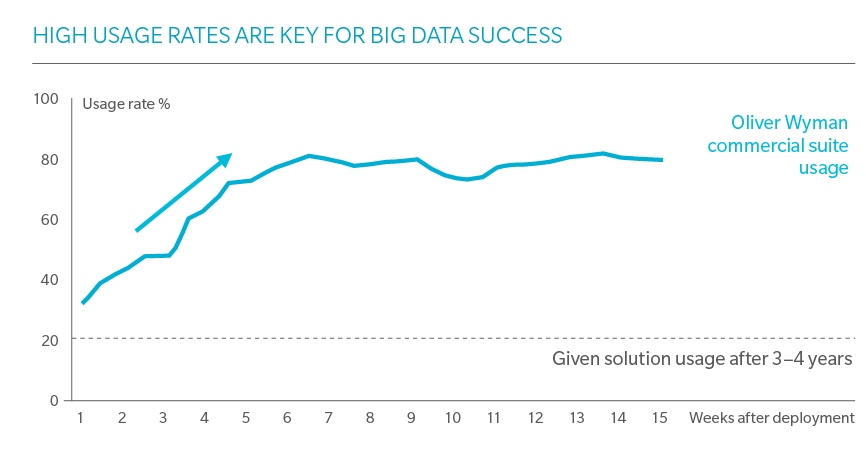
Intuitive And Attractive
Systems need to be intuitive and attractive to use. Successful smartphone apps offer easy usability even if they are highly complex. What these apps have in common is that they deliberately discard everything that is not absolutely necessary. This needs to be done in corporate environments, too. Oliver Wyman for example has developed app-style systems for a range of commercial decision-making. In development, User Experience Designers test and optimize every user interaction to create applications that are so simple they require no instructions. One example is promotional decisions on both the wholesale and retail levels. By analyzing various sales promotions (such as promotion type, discount level, region, dealer, communication channel), their effectiveness can be predicted. Via a promotion app, the salesperson can then simulate a certain promotion that has been planned. The app will show the salesperson the expected rise in units and financial income, discount spend, cannibalization, and resulting net effects. Applications also need to be promoted within the organization to create a pull-effect by advertising their benefits. Convinced users will act as advocates by sharing their experiences with wider audiences.